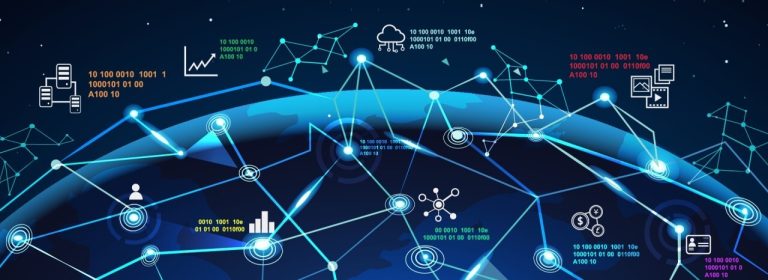
Data analytics is the systematic computational analysis of data to extract meaningful insights and inform decision-making processes. This article delves into the fundamentals, types, applications, benefits, challenges, and future trends of data analytics, highlighting its transformative impact across industries and sectors.
Fundamentals of Data Analytics
Data analytics involves the process of examining raw data to draw conclusions about the information it contains. Key components include:
- Data Collection: Gathering structured or unstructured data from various sources, including databases, sensors, social media, and internet of things (IoT) devices.
- Data Cleaning and Preparation: Preparing data by removing inconsistencies, handling missing values, and transforming data into a usable format for analysis.
- Data Analysis: Applying statistical and mathematical techniques, machine learning algorithms, and data visualization tools to uncover patterns, trends, and correlations within the data.
- Insights and Decision-Making: Deriving actionable insights that support informed decision-making, strategy formulation, and operational improvements.
Types of Data Analytics
- Descriptive Analytics: Using historical data to summarize and describe past events, trends, and patterns. It answers the question “What happened?”
- Diagnostic Analytics: Analyzing data to understand the reasons behind past outcomes or events. It answers the question “Why did it happen?”
- Predictive Analytics: Utilizing statistical models and machine learning algorithms to forecast future outcomes based on historical data. It answers the question “What is likely to happen?”
- Prescriptive Analytics: Recommending actions or strategies to optimize outcomes based on predictive analytics and decision-making rules. It answers the question “What should we do?”
Applications of Data Analytics
- Business Intelligence: Analyzing sales data, customer behavior, and market trends to improve marketing strategies, customer segmentation, and operational efficiency.
- Healthcare: Using patient data and medical records to predict disease outbreaks, personalize treatments, and optimize hospital operations.
- Finance: Analyzing financial transactions, market trends, and risk factors to make informed investment decisions, detect fraud, and manage financial portfolios.
- Manufacturing and Supply Chain: Optimizing production processes, inventory management, and logistics through real-time data analysis and predictive maintenance.
Benefits of Data Analytics
- Informed Decision-Making: Empowering organizations to make data-driven decisions based on insights derived from analytics.
- Operational Efficiency: Streamlining processes, reducing costs, and improving productivity through data-driven optimizations.
- Competitive Advantage: Gaining insights into market trends, customer preferences, and emerging opportunities to stay ahead of competitors.
- Personalization and Customer Experience: Tailoring products, services, and marketing campaigns to meet the needs and preferences of individual customers.
Challenges in Data Analytics
- Data Quality and Integration: Ensuring data accuracy, completeness, and consistency across different sources and formats.
- Privacy and Security: Protecting sensitive data from breaches, unauthorized access, and compliance with data protection regulations (e.g., GDPR, CCPA).
- Skill Shortage: Addressing the shortage of skilled data scientists, analysts, and professionals proficient in data analytics tools and techniques.
- Ethical Considerations: Managing biases in data analysis, ensuring fairness, transparency, and ethical use of data in decision-making processes.
Future Trends in Data Analytics
- Artificial Intelligence and Machine Learning: Advancing AI algorithms for automated data analysis, pattern recognition, and predictive modeling.
- Big Data and IoT Integration: Leveraging massive volumes of data generated by IoT devices for real-time analytics and insights.
- Edge Analytics: Performing data analysis at the edge of the network, closer to the data source, to enable faster decision-making and reduce latency.
- Data Democratization: Empowering non-technical users with self-service analytics tools and platforms to access and analyze data independently.
Conclusion
Data analytics is a powerful tool that transforms raw data into valuable insights, driving informed decision-making, operational efficiencies, and competitive advantages across industries. As organizations continue to harness the potential of data analytics, addressing challenges such as data quality, privacy concerns, and skill gaps will be crucial. Embracing emerging technologies, fostering a data-driven culture, and prioritizing ethical considerations will pave the way for leveraging data analytics to its fullest potential in shaping the future of businesses, industries, and society as a whole.